In my first two articles on Strokes Gained, I covered two of my three biggest issues with its use as a data source for daily fantasy sports. The articles focused on explaining why strokes gained/ShotLink data doesn’t have as large of an edge over properly adjusted conventional data as is commonly held. That being said, I think ShotLink will always be better than conventional data if both are available. From a strictly mathematical definition, ShotLink is conventional data plus additional information. That additional information can only help our decisions, and the transitive property takes care of the rest.
The problem with ShotLink data is that it’s not always available: Non-PGA tours (and even some PGA events) don’t have any ShotLink data. You might think the missing data doesn’t amount to much. Who cares if we’re missing a couple results for the Bernd Wiesbergers and Shane Lowrys of the world? There’s not a lot of Euros on tour regularly, so isn’t this just a rounding error for a couple of players we wouldn’t roster anyway? Unfortunately, that’s not the case. We’ll explore exactly how big these data gaps are, who they effect, and what their practical impact is, and then we’ll examine some of the ways to fill them.
Before we get into the details, I want to cover how truly insane outright missing data is from a DFS perspective. Imagine during football season that a game between two low-rated teams like the Jaguars and Browns had such little interest that there wasn’t even a box score to be found, only the final score of the game. Or if the Sixers went on a five-game West Coast trip, but you had no idea what each player’s points, rebounds, or even minutes were during those games. Seriously, what would you do during your research process? Would you pretend those games just didn’t happen? Or would you try your best to get some useful information out of those results?
In PGA, apparently most people prefer the former. It’s understandable: Most people developed their DFS research habits in other sports, and other sports just don’t have this problem. You don’t see the Gasol brothers playing a quarter of the season for FC Barcelona or Alex Ovechkin taking a three-week holiday in the KHL. If they did, would you still go to NBA.com and take the Gasols’ NBA-only stats at face value? Or would you collect their Euroleague box scores and try to figure out if there’s additional useful information? Honestly, I still feel like plenty of people would choose the first option. Combining the two would require grabbing data from different sources, developing a method to translate the results into a common form, and understanding how to weight them appropriately. That’s a decent amount of work for a small subset of players who are “only” playing a quarter of their games overseas. You could easily talk yourself into deciding that it’s just not worth the effort.
So how well does that analogy hold up in PGA? First, we need to decide on some threshold for when players have a “significant” amount of non-ShotLink data in their history: 20 percent of tournaments played is a decent threshold for me. I could see an argument for 25 to 30 percent, but given the importance of recent form my standards for missing data are a little tighter. I went back and looked at every PGA event in 2015 and filtered the fields to include players with at least eight events previously played in the past 75 weeks. I calculated the fraction of their 75-weeks-prior game log that had ShotLink data and plotted the distribution of those percentages. Here’s what the distribution looks like:
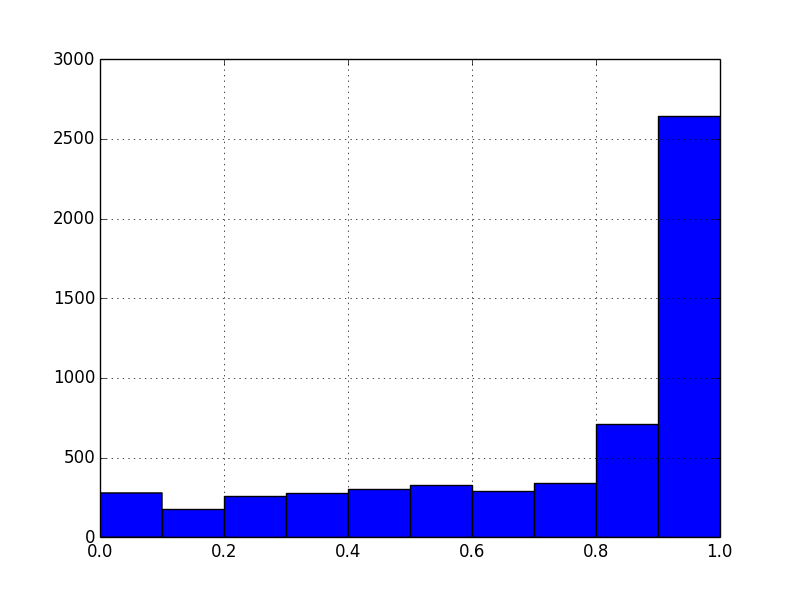
Most players are bunched on the right, indicating that the solid majority of golfers play over 80 percent of their events on the PGA tour. However, the tail isn’t small enough to ignore. In total, 2,256 of the 5,611 rosterable players in 2015 (40 percent) had at least 20 percent of their results from non-ShotLink tournaments at the time of their event. Think about that: on average, two out of five golfers in a given PGA slate have significant gaps in their ShotLink data.
Okay, but who exactly are these players that have these gaps? If the high-gap players are all concentrated at the low end of the salary range and aren’t very good players, you don’t care about them anyway, since you’re not likely to roster them. Here’s a scatter plot of each player’s normalized long-term adjusted round score (a proxy for salary) versus their ShotLink data ratio:
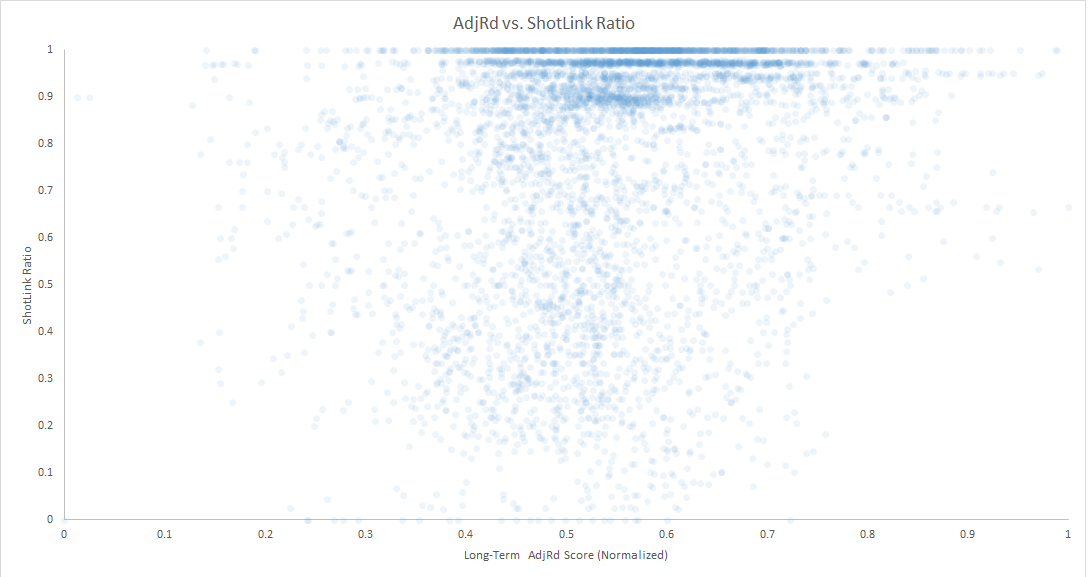
If the high-gap players were highly concentrated among low salaries, you would see the dots cluster more towards the left in the lower half of that plot. However, the scatter in the lower half is pretty uniform from left to right, indicating that there are high-gap players across all levels of salary.
If push comes to shove, you could still roster a player if you only had his ShotLink results. But why would you willingly exclude helpful additional information? And it’s not just any additional information: It’s stuff that, by and large, other DFS players are not incorporating, making it very high-leverage information. It’s hard enough to find value plays in DFS golf — finding value plays that no one else knows about is even harder. Incorporating non-ShotLink results has a compounding effect on finding those “hidden” value plays. Not only are other DFS players not going to include those results, but those high-value picks you find will be highly concentrated among non-household names, increasing the likelihood that those picks will have low ownership.
There are different approaches to incorporating non-ShotLink events, each with their relative merits and flaws. In my opinion, the cleanest and most straightforward approach is using only properly adjusted conventional data for analysis. The tours can be combined seamlessly, you don’t have to worry about the accuracy of proxy fills, and overlapping metrics are kept to a minimum. That’s why you won’t find any Strokes Gained data in Player Models. It was a deliberate choice on our part to balance accuracy and simplicity of the modeling process. Having a more complex integration process may have a place in pure projection systems, but for the purposes of empowering our users to make data-driven decisions, ShotLink/Strokes Gained has too many gaps and doesn’t bring enough value-added to warrant inclusion.